The Future of Autonomous Finance: AI Agents, Big Data, and the Shift from GUIs to NLIs
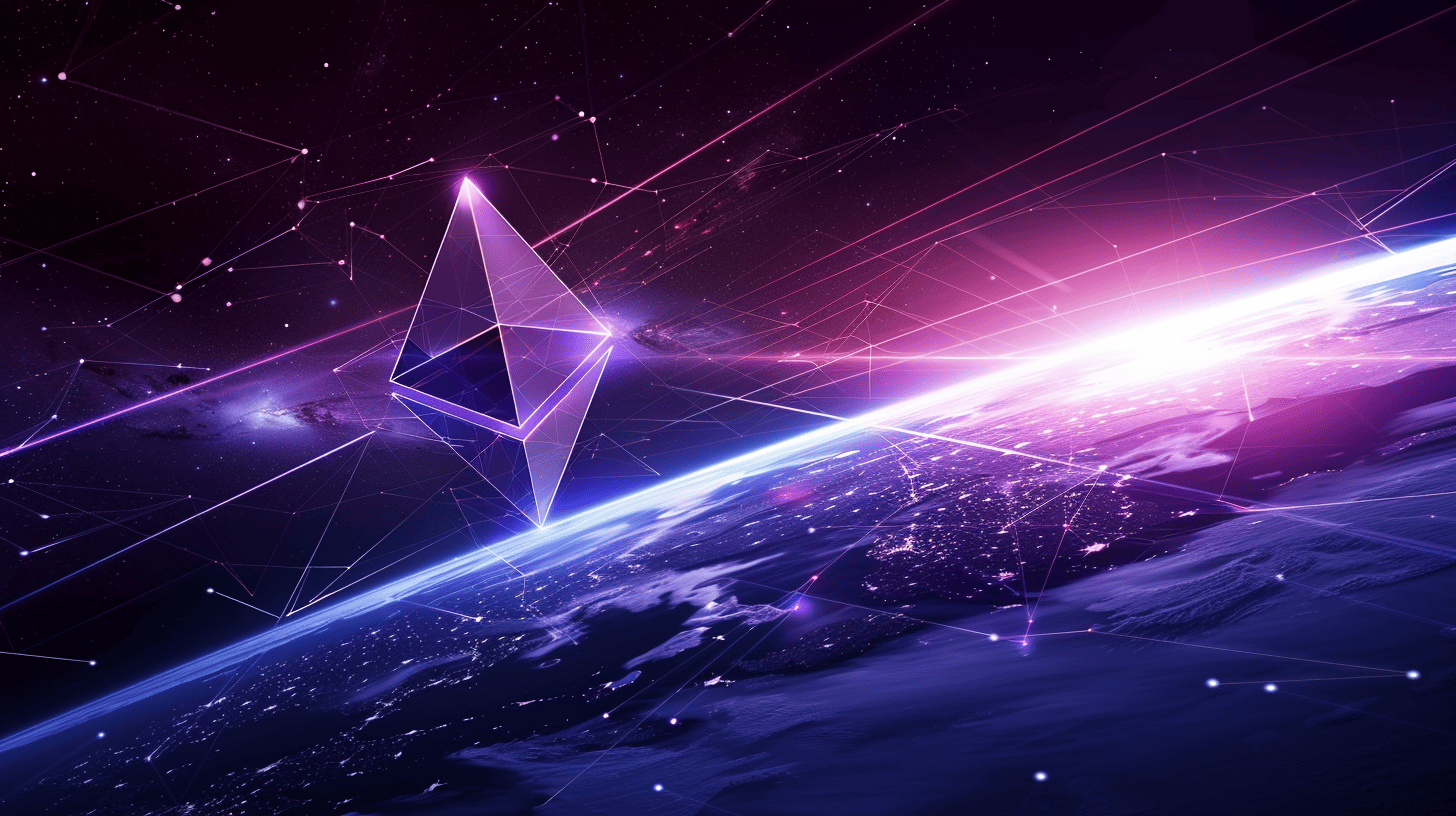
Crypto’s ChatGPT Moment?
The financial landscape is undergoing a profound shift, with artificial intelligence (AI) and big data driving the next wave of innovation in autonomous finance. Just as ChatGPT revolutionized AI adoption by introducing natural language interfaces (NLIs) that made advanced machine learning accessible to everyone, crypto and decentralized finance (DeFi) are now experiencing their own ChatGPT moment.
Instead of navigating complex graphical user interfaces (GUIs), manually tracking liquidity pools, or spending hours researching DeFi strategies, users can now interact with autonomous AI agents via simple natural language commands. These AI-driven NLIs, powered by deep learning and on-chain analytics, translate user intents into optimized financial strategies, risk assessments, and automated execution — all in real-time.
At the forefront of this transformation is a new class of AI-powered financial agents, known as Autonomous Linguistic Financial Agents (ALFAs). These intelligent agents can analyze billions of on-chain transactions, track Smart Money movements, monitor social sentiment, and execute high-level trading strategies — all autonomously.
The rise of AI-native finance will reshape crypto trading, investment management, and risk assessment, marking a paradigm shift in how financial markets operate.
The Problem: Data Overload and Fragmentation in Web3
The Web3 ecosystem is decentralized, transparent, and fast-moving. Every transaction, liquidity shift, governance vote, and market trend is recorded on-chain or discussed across social platforms. However, this abundance of data creates more noise than signal, making it incredibly difficult for traders, investors, and institutions to extract real-time actionable intelligence.
Unlike traditional finance — where structured reports and centralized market data simplify decision-making — Web3 data is highly fragmented across multiple chains, protocols, and social media channels.
Challenges of Traditional Web3 Market Analysis
-
On-Chain Complexity
Billions of transactions occur across Ethereum, Solana, Arbitrum, BSC, and Layer 2s. Tracking whale movements, token migrations, and DEX liquidity pools is a manual, time-consuming process. -
Off-Chain Market Sentiment
Crypto moves at the speed of social consensus. Telegram, Twitter (X), Discord, and governance forums drive market shifts, yet current analytics tools struggle to extract meaningful insights from billions of unstructured messages. -
High-Frequency Market Dynamics
Thousands of new tokens, liquidity pools, and governance proposals emerge daily, requiring instant AI-driven assessments. -
Complex User Interfaces
Traditional GUIs require extensive financial literacy, technical knowledge, and manual execution of multi-step transactions.
The solution? AI-powered NLIs and fully autonomous financial agents that eliminate complexity, consolidate workflows, and optimize decision-making.
AI Agents: The Evolution of Web3 Market Intelligence
The next generation of financial intelligence is being built on AI-powered autonomous agents, which act as intermediaries between human intent and decentralized execution. These agents continuously analyze on-chain data, off-chain sentiment, and trading signals, allowing users to make faster, smarter financial decisions — or even allowing AI agents to act completely autonomously.
What Makes AI Agents Different from Traditional Bots?
A true autonomous financial AI agent is far more advanced than an ordinary trading bot. It must:
- Perceive on-chain and off-chain data in real-time.
- Reason and adapt, making decisions based on incomplete or evolving information.
- Simulate market scenarios and optimize yield strategies, portfolio allocations, and risk models.
- Proactively execute transactions, rather than waiting for user input.
- Collaborate with other AI agents, enabling decentralized financial ecosystems to operate autonomously.
Instead of just executing predefined rules, AI-driven ALFAs can continuously learn, adapt, and generate optimal financial strategies.
The AI-Driven Financial Tech Stack
For AI-powered financial automation to work at scale, a multi-layered AI architecture is required. RateX.ai and other emerging AI finance platforms operate on a four-layer intelligence stack, which integrates real-time blockchain data, advanced machine learning, and automated execution models.
Data Synthesis & Aggregation
AI agents ingest real-time data from both on-chain and off-chain sources, including:
-
On-chain data: Smart contract interactions, token movements, liquidity pools, whale transactions.
-
Market feeds: Aggregated CEX + DEX price data, funding rates, open interest.
-
Social sentiment: NLP-powered sentiment analysis of Twitter (X), Telegram, and governance discussions.
Adaptive Machine Learning Models
Once data is collected, AI-driven adaptive models process it using:
- Reinforcement learning algorithms to optimize trading/yield farming strategies.
- Transformer-based NLP models to extract signals from social sentiment clusters.
- LSTM networks & Graph Neural Networks (GNNs) for predictive price modeling.
Real-Time Market Intelligence & Strategy Optimization
AI-driven inference models convert raw data into real-time intelligence:
- AI-driven token scoring: Automated fundamental & technical analysis.
- Risk assessments: Automated alerts for liquidity risks, market manipulations, and governance changes.
- Dynamic rebalancing: AI models continuously optimize capital allocations based on real-time conditions.
Execution & Action Orchestration
Once a strategy is optimized, the AI agent executes transactions autonomously:
- Deploys capital across multiple yield farms, lending protocols, and LP pools.
- Executes automated arbitrage strategies across CEXs & DEXs.
- Dynamically rebalances portfolios based on risk models and market sentiment.
This AI-driven automation eliminates human inefficiencies, allowing traders and institutions to react to market opportunities instantly.
The Future: AI-Native Agentic Economies
Beyond individual AI agents, a new economic model is emerging — agentic economies. These are decentralized ecosystems where swarms of AI agents interact autonomously, optimizing liquidity, executing trades, and managing risk without human intervention.
For example, in an AI-driven DeFi protocol, multiple specialized agents could collaborate:
- A forecasting agent predicts short-term price action.
- A trading agent executes orders based on forecasted movements.
- A liquidity management agent adjusts Uniswap v4 LP positions using real-time market conditions.
By working together in self-optimizing closed-loop systems, these agents can outperform human traders and drive markets toward greater efficiency.
Building Trust in AI Finance
As AI agents handle more complex financial operations, trust and security become critical. Technologies like zero-knowledge proofs (ZKPs) and verifiable AI inference will ensure AI models operate transparently and securely, preventing manipulation or bias.
Conclusion: The AI-Native Future of Finance
The shift from GUIs to NLIs, and from manual execution to AI-driven autonomous finance, represents the biggest transformation in crypto since the launch of DeFi. AI-powered agents will redefine how traders, investors, and institutions interact with Web3 markets, providing instant, optimized financial decision-making with minimal friction.
Just as ChatGPT unlocked mass adoption of AI, crypto’s ChatGPT moment is arriving — where natural language AI interfaces will make autonomous finance accessible to all.
🚀 Ready to experience the future of AI-native DeFi?
Schedule a demo with RAYS today.